Explanation and analysis¶
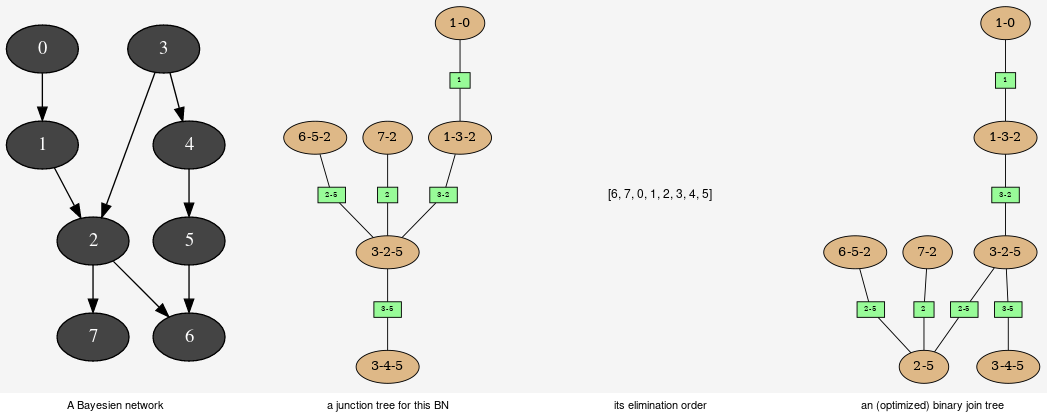
This tools aimed to provide some different views on the Bayesian network in order to explore its qualitative and/or quantitave behaviours.
- class pyAgrum.JunctionTreeGenerator¶
JunctionTreeGenerator is use to generate junction tree or binary junction tree from Bayesian networks.
- JunctionTreeGenerator() -> JunctionTreeGenerator
default constructor
- binaryJoinTree(*args)¶
Computes the binary joint tree for its parameters. If the first parameter is a graph, the heurisitcs assume that all the node have the same domain size (2). If given, the heuristic takes into account the partial order for its elimination order.
- Parameters
g (pyAgrum.UndiGraph) – a undirected graph
dag (pyAgrum.DAG) – a dag
bn (pyAgrum.BayesNet) – a BayesianNetwork
partial_order (List[List[int]]) – a partial order among the nodeIDs
- Returns
the current binary joint tree
- Return type
- eliminationOrder(*args)¶
Computes the elimination for its parameters. If the first parameter is a graph, the heurisitcs assume that all the node have the same domain size (2). If given, the heuristic takes into account the partial order for its elimination order.
- Parameters
g (pyAgrum.UndiGraph) – a undirected graph
dag (pyAgrum.DAG) – a dag
bn (pyAgrum.BayesNet) – a BayesianNetwork
partial_order (List[List[int]]) – a partial order among the nodeIDs
- Returns
the current elimination order.
- Return type
- junctionTree(*args)¶
Computes the junction tree for its parameters. If the first parameter is a graph, the heurisitcs assume that all the node have the same domain size (2). If given, the heuristic takes into account the partial order for its elimination order.
- Parameters
g (pyAgrum.UndiGraph) – a undirected graph
dag (pyAgrum.DAG) – a dag
bn (pyAgrum.BayesNet) – a BayesianNetwork
partial_order (List[List[int]]) – a partial order among the nodeIDs
- Returns
the current junction tree.
- Return type
- class pyAgrum.EssentialGraph(*args)¶
Class building the essential graph from a BN.
Essential graph is a mixed graph (Chain Graph) that represents the class of markov equivalent Bayesian networks (with the same independency model).
- EssentialGraph(m) -> EssentialGraph
- Parameters:
m (pyAgrum.DAGmodel) – a DAGmodel
- arcs()¶
- Returns
The lisf of arcs in the EssentialGraph
- Return type
list
- children(id)¶
- Parameters
id (int) – the id of the parent
- Returns
the set of all the children
- Return type
Set
- connectedComponents()¶
connected components from a graph/BN
Compute the connected components of a pyAgrum’s graph or Bayesian Network (more generally an object that has nodes, children/parents or neighbours methods)
The firstly visited node for each component is called a ‘root’ and is used as a key for the component. This root has been arbitrarily chosen during the algorithm.
- Returns
dict of connected components (as set of nodeIds (int)) with a nodeId (root) of each component as key.
- Return type
dict(int,Set[int])
- edges()¶
- Returns
the list of the edges
- Return type
List
- mixedGraph()¶
- Returns
the mixed graph
- Return type
- neighbours(id)¶
- Parameters
id (int) – the id of the checked node
- Returns
The set of edges adjacent to the given node
- Return type
Set
- nodes()¶
- Return type
object
- parents(id)¶
- Parameters
id (
int
) – The id of the child node- Returns
the set of the parents ids.
- Return type
Set
- size()¶
- Returns
the number of nodes in the graph
- Return type
int
- sizeArcs()¶
- Returns
the number of arcs in the graph
- Return type
int
- sizeEdges()¶
- Returns
the number of edges in the graph
- Return type
int
- sizeNodes()¶
- Returns
the number of nodes in the graph
- Return type
int
- toDot()¶
- Returns
a friendly display of the graph in DOT format
- Return type
str
- class pyAgrum.MarkovBlanket(*args)¶
Class building the Markov blanket of a node in a graph.
- MarkovBlanket(m,n) -> MarkovBlanket
- Parameters:
m (pyAgrum.DAGmodel) – a DAGmodel
n (int) – a node id
- MarkovBlanket(m,name) -> MarkovBlanket
- Parameters:
m (pyAgrum.DAGmodel) – a DAGmodel
name (str) – a node name
- arcs()¶
- Returns
the list of the arcs
- Return type
List
- children(id)¶
- Parameters
id (int) – the id of the parent
- Returns
the set of all the children
- Return type
Set
- connectedComponents()¶
connected components from a graph/BN
Compute the connected components of a pyAgrum’s graph or Bayesian Network (more generally an object that has nodes, children/parents or neighbours methods)
The firstly visited node for each component is called a ‘root’ and is used as a key for the component. This root has been arbitrarily chosen during the algorithm.
- Returns
dict of connected components (as set of nodeIds (int)) with a nodeId (root) of each component as key.
- Return type
dict(int,Set[int])
- dag()¶
- Returns
a copy of the DAG
- Return type
- hasSameStructure(other)¶
- Parameters
pyAgrum.DAGmodel – a direct acyclic model
- Returns
True if all the named node are the same and all the named arcs are the same
- Return type
bool
- nodes()¶
- Returns
the set of ids
- Return type
set
- parents(id)¶
- Parameters
id (
int
) – The id of the child node- Returns
the set of the parents ids.
- Return type
Set
- size()¶
- Returns
the number of nodes in the graph
- Return type
int
- sizeArcs()¶
- Returns
the number of arcs in the graph
- Return type
int
- sizeNodes()¶
- Returns
the number of nodes in the graph
- Return type
int
- toDot()¶
- Returns
a friendly display of the graph in DOT format
- Return type
str